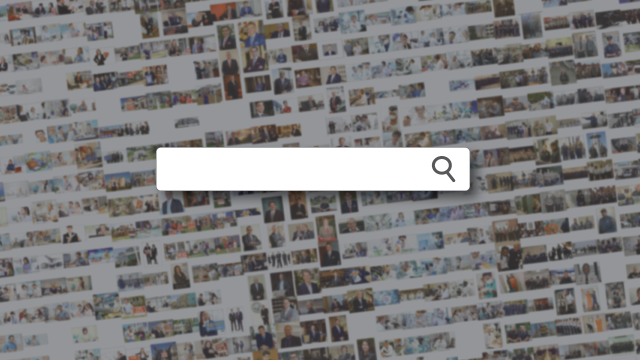

Online media organizations, social media sites and individuals add vast quantities of images to the web each day. These images can then appear in search engines as users look for pictures representing common phrases or topics. Because the way that men and women are represented in these online search results might be connected to the way people understand gender and society, some academic researchers have specifically focused on the ways women and men are depicted in the workplace in online images.
A new Pew Research Center study extends this line of research by using a computational method – machine vision to analyze a broad sample of images from Google Image Search that depict men and women working common jobs, and then comparing those results with real-world data about the gender composition of the U.S. workforce. The study finds that the share of each gender pictured varies widely across the spectrum of careers tested. But in the majority of jobs examined, women are somewhat underrepresented in online images relative to their actual participation rates in those jobs in the United States, based on 2017 Bureau of Labor Statistics data. Across all individuals shown in the search results, men appear 60% of the time. And, when women appear, they appear lower in the search results than men.
To conduct this analysis, Pew Research Center analyzed over 10,000 images appearing in U.S.-based, English-language search results for 105 common occupations.1 The jobs analyzed in the study include everyday occupations such as hair stylist, librarian, butcher and plumber, among many others. Researchers used a machine vision algorithm to estimate whether each person appearing in a given image was male or female. Next, they calculated the estimated percentages of men and women depicted in the top 100 Google Image Search results for each of those jobs to assess whether the results reflected the actual percentages in each occupation who are men and women.
Key findings of the analysis include:
- Image results for common job searches somewhat overrepresent men relative to women. Across the sample of image searches for 105 jobs, an estimated 60% of the individuals that appeared in the image results were men (40% were women). According to Bureau of Labor Statistics (BLS) data, men made up 54% of all individuals employed in these jobs.
- For more than half the tested job categories, images appearing in searches underrepresent women relative to their actual participation in those jobs, according to federal data. When compared with BLS data measuring the actual share of each profession’s workforce that is male and female, women were underrepresented in search results for 57% of the 105 jobs we analyzed. They were overrepresented in 42%.2
- The underrepresentation of women was concentrated in a few jobs. Across the 10 jobs where women were the most underrepresented in image searches, the average rate they appeared was 33 percentage points lower than the actual rate at which they held those jobs. Across the other 50 jobs where women were underrepresented, the average rate they appeared was just 12 points lower than their real labor force participation rate.
- Images containing women appear further down the page in search results for many jobs. Images depicting women engaged in jobs tended to appear lower in search results compared with men, no matter their actual share in the labor force. On average, the first image containing a woman appeared about four images from the first result, while on average the first image containing a man appeared two images from the top.
- Image search results display more gender diversity than actually exists, on average. According to the BLS, 38% of jobs in the study are predominantly held by either men or women (defined as jobs with 80% or more male or female workers). However, across image searches for all jobs, only 21% showed predominantly men or women.
When internet users search for images, a complex mix of factors affects what they see. Content searchers and content creators alike can influence which images draw the most attention. But researchers do not have direct access to the underlying image selection process, nor do we know how much any one factor matters when a search algorithm decides what images to select. Search algorithms are often designed to highlight images that the algorithms “expect” will be most relevant for a particular user based on their search history or other browsing behavior. But image creators and other users also can act in ways that at least partially determine the rate at which certain images appear. Algorithms are capable of learning from both content consumers and content producers. So, for example, if content producers often use men to illustrate the term “chief executive,” algorithms might preserve or magnify these tendencies. At the same time, the ways that internet users click on particular images might make those images appear more relevant, and thus more likely to be selected by the algorithm. Since algorithms for image search are proprietary, the Center’s research team could not evaluate them directly in this analysis.
To ensure that the images analyzed in the study depicted people at work in the jobs being searched, researchers checked whether or not the images returned in search results actually showed individuals engaged in the particular occupation of interest. Many searches for jobs did not yield a majority of images actually depicting people working in those jobs. Researchers examined each set of images and found that 44% of image search terms from a broader list of initial occupations returned a majority of images that showed people engaged in the job – rather than other people or objects associated with the job. In other words, for just 106 of the initial list of 239 jobs did the majority of images associated with each job depict the relevant worker.
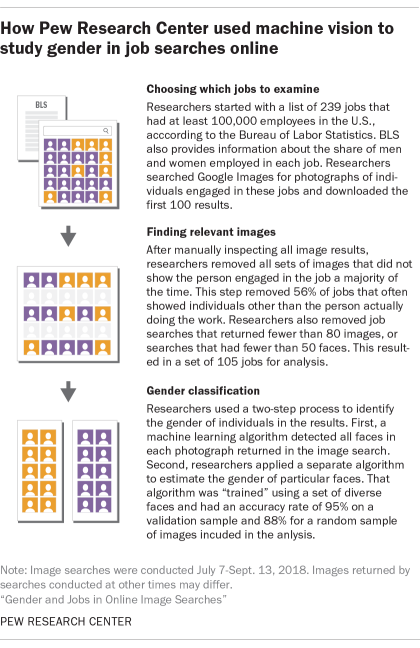
The remaining 56% of searches returned images that did not reliably show a person associated with the searched occupation: Some showed clients or customers, rather than practitioners of the occupation, or depicted non-human objects. These images were not included in the analysis. For example, the majority of image results for the term “physical therapist” showed individuals receiving care rather than individuals engaging in the duties associated with being a physical therapist (see Methodology for additional details). Finally, researchers removed searches for jobs that returned fewer than 80 total images, resulting in an analysis sample of 105 sets of job-relevant images.
The image searches were conducted between July 7 and Sept. 13, 2018, and the specific images examined here may no longer appear. Images with multiple individuals were included in the analysis, and each individual was analyzed separately.
How researchers estimated gender using machine vision
To estimate the gender of individuals in images, this analysis relies on deep learning techniques from the field of computer vision, which focuses on algorithms that identify different objects in images. Researchers used a method called “transfer learning” to repurpose an existing algorithm to the task of recognizing male and female faces.
To train a machine learning system to “learn” the facial traits associated with male and female faces, researchers assembled a set of images that humans had identified as male or female. Previous research has found that image classification algorithms available through commercial vendors suffer from inconsistent accuracy across racial groups. Accurate gender classification across racial groups is especially important in this context because researchers also used the model to classify results across different countries and languages in a separate analysis. One limitation of the machine vision model used here is that it cannot identify nonbinary individuals, since the training data included only images labeled as male or female. In addition, the model’s estimate of gender is based on physical appearance, not how individuals actually identify.
The research team assembled a large and diverse set of images to train the model. Researchers used 26,981 labeled faces from different image sources to achieve a relatively balanced demographic sample from which to train the algorithm. This dataset included individuals from different countries and minority groups. The training images vary in size and image quality, which helped ensure that the classifier would work across many different kinds of images. The training images were also randomly rotated and clipped to maintain accuracy across photos in many orientations.
The gender classification model was trained with 80% of the human-labeled data, and then tested on the remaining 20% of those images. It achieved 95% accuracy on the set of images that were not used to train the model. The model’s performance was broadly consistent across different minority groups (see Methodology).
After researchers tested the model’s accuracy, they began applying it to search queries for particular kinds of jobs. Researchers imposed filtering criteria on the queries, in addition to checking whether the images showed individuals engaged in the job. First, researchers applied Google’s “photo” filter, which is meant to remove animated or computer-generated images from search results. Second, researchers removed all the job searches with fewer than 80 images overall, or with fewer than 50 images containing human faces in the results. See Methodology for additional details.
As a final validation step, researchers tested the model on a sample of actual Google Image Search results, as classified by human coders. Across a random sample of 996 images from those included in the analysis, the model had an 88% accuracy rate. Although 1,000 images were randomly selected, four either had a dead link or were not coded by three coders.
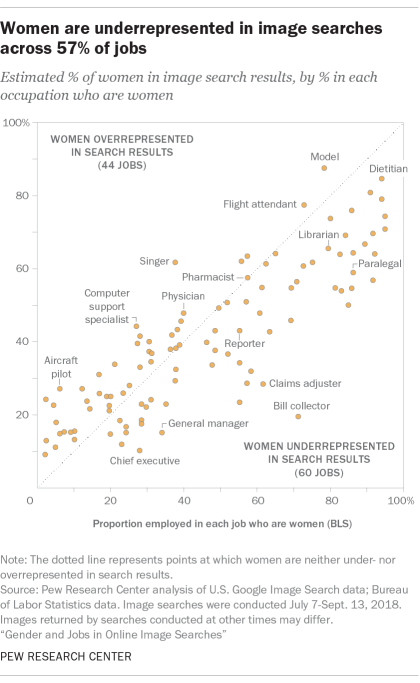
Across the 105 occupations included in the analysis, 40% of the individuals depicted across all search results were women. And for some kinds of jobs, that rate was much lower: Women appeared in search results for chief executive at a rate of 10%, and at a rate of 15% across results for general manager.
These results understate the degree to which women actually hold these jobs in the U.S. According to data from the Bureau of Labor Statistics, 28% of people employed as chief executives are women, as are 34% of those employed as general managers.3 Across all jobs, the rate at which women appear in image searches was 6 percentage points lower than the rate at which they actually hold those jobs.
For 16% of job searches (notwithstanding overall rates of representation, which include any degree of over- or underrepresentation), the results were relatively close to the actual share of women holding those jobs – that is, the results were within 5 percentage points of their actual share of the workforce in that occupation.
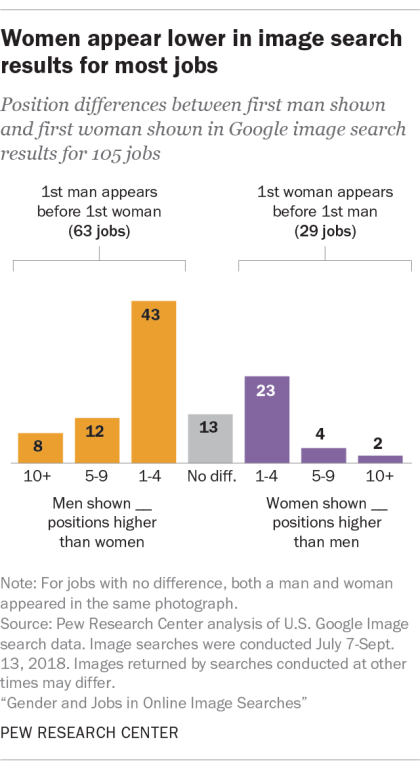
Even in jobs where women were only slightly over- or underrepresented, there are disparities in where images showing women appear within the search results. Researchers found that the average search position of an image depicting a woman tends to be lower in search results compared with images depicting a man. The higher the search position (1 being the highest, 100 the lowest), the earlier it appears in the set of images returned by Google Image Search (position is represented left to right, top to bottom). For this sample of jobs, the average image position of the first woman shown is 3.7, compared with 2.0 for the first man.
To use a concrete example, the image search for head cook (which slightly overrepresented women overall) showed the first woman in the fifth position, while a man appeared in the first position. For engineering technician (which underrepresented women by 5 percentage points), the first woman appeared in the 11th position, while the first man appeared in the first position.
The study also found that image searches for certain gender-dominant jobs had more gender diversity relative to the actual rate at which men and women held those jobs. Researchers classified a job as predominantly held by men or women if at least 80% of those employed in the occupation were either men or women. Overall, 38% of these 105 jobs are predominantly held by men or women, according to BLS data. But when it came to image results for the same 105 jobs, just 21% predominantly featured men or women, using the same 80% threshold. In other words, image results for some predominantly male or female jobs are more gender diverse than would be expected if they perfectly matched the actual rate at which men and women held those jobs.
Examples of image searches that predominantly depict men include general manager and announcer, while in fact these jobs are more often held by women: 34% of general managers are women, but only 15% of individuals in search results for that occupation are women. Similarly, 23% of announcers are women, but 12% of people in search results for “announcer” are women. But for jobs like plumber, machinist and truck driver, which are predominantly held by men, image results show women at rates of 9%, 11% and 15%, respectively. These rates are substantially higher than the actual number of women who work as plumbers (2%), machinists (5%) and truck drivers (6%).
Gendered depictions of some jobs are consistent across countries
A separate Pew Research Center analysis approximated job-related searches for 18 different countries: Argentina, Australia, Brazil, Canada, France, Germany, India, Indonesia, Italy, Japan, Mexico, Russia, Saudi Arabia, South Africa, South Korea, Turkey, the United Kingdom and the United States. These countries were selected because they are members of the Group of 20, and together, their economies account for 63% of the global economy.4
Researchers obtained translations of the top 100 occupations (according to U.S. employment figures) for each of 12 languages, using either the official language of each country or the most prevalent language (if there were multiple official languages). Next, the team restricted the list to occupations that had relevant search results across a wide set of country-language combinations, using the same criteria as the U.S. analysis. For languages with gendered terms for different jobs, researchers used the male form of occupation titles when that form was the generic way to reference people of unknown gender employed in that job. For example, in Spanish, “cocinero” is a generic reference to a chef and is the masculine form of the noun. See the Methodology for additional details about the global analysis and translation process.5
Researchers set custom search parameters for each language and corresponding country, and then collected up to 100 images for each county-language combination.6 Overall, researchers queried a total of 30,236 images for 307 translated job titles across the 18 countries. Some key findings of this analysis include:
- The association between gender and certain kinds of jobs is global. Jobs such as housekeeper, customer service representative and nurse had the highest estimated percentage of women across all languages, whereas CEO, clergy and professor had the lowest estimated percentage of women across languages, on average. Searches for nurses, for example, showed women 84% of the time in Japanese results and 80% of the time in English-language searches in the UK. Image searches for professor showed women 19% of the time for Hindi (India), compared with 22% for German (Germany).
- Images that show women appeared lower in search results than images that show men. The first image that showed a woman in searches for CEO or professor averaged a position of about 16 and 13, respectively, across all country-language combinations, compared with an image position of about 2 for men in both jobs. For housekeeper and customer service representative, images that depict women appear earlier in the results than those that depict men.
- A lack of real-world comparison data for occupations at the global level limits the conclusions we can make. Because researchers were not able to obtain parallel, real-world data about the percentages of men and women working in each job for all countries, it was not possible to conclude whether the image results overrepresent or underrepresent women in each occupation and country.
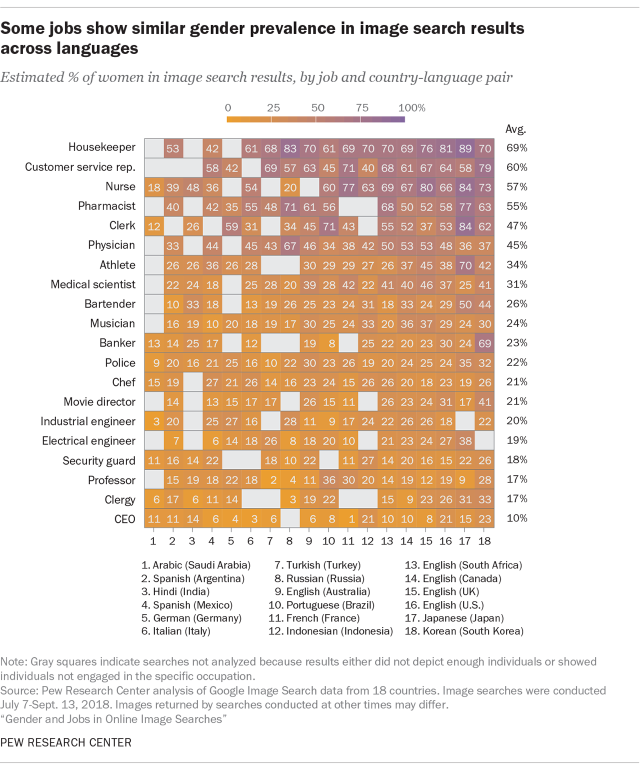
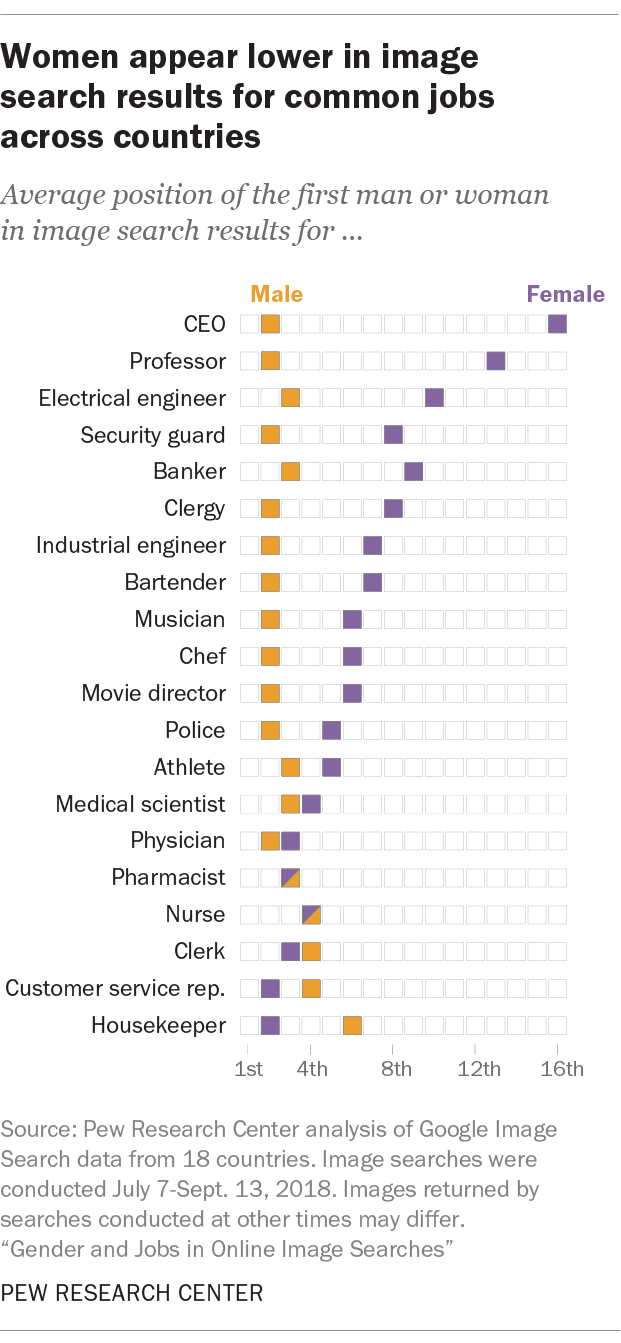